Probability, causality, and how they impact responsibility
Probability, causality, and how they impact responsibility
“Artificial intelligence” algorithms are probabilistic and lack causality. Can we still call them “intelligent”? and can we trust them to make decisions?
The smoking gun
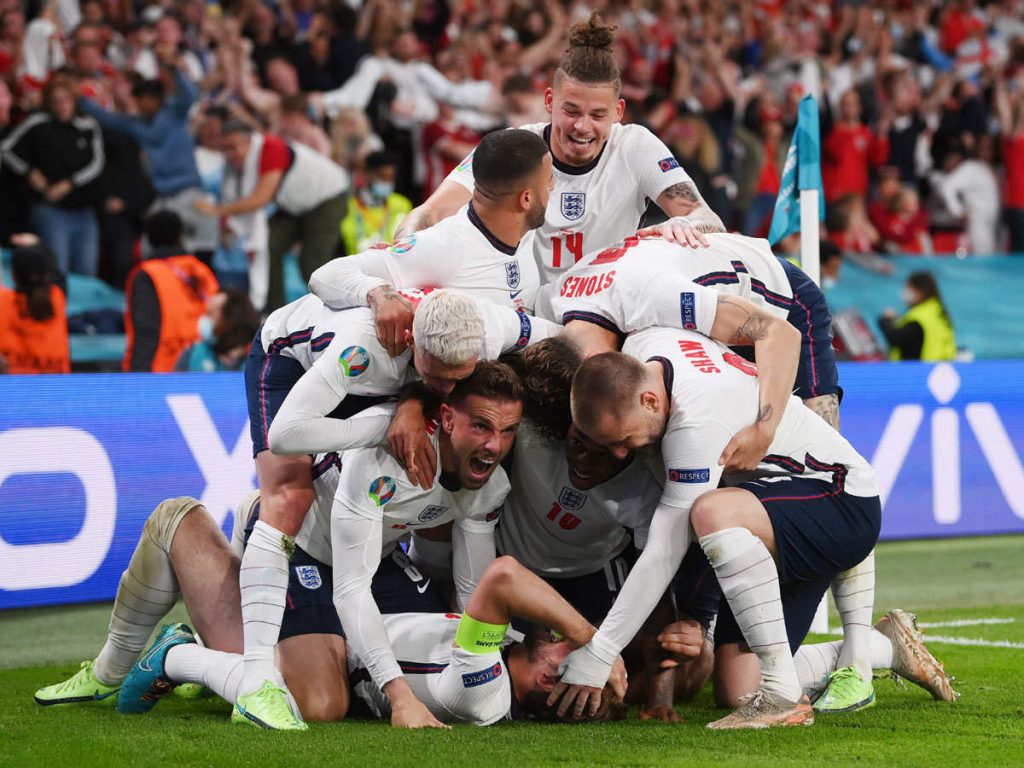
England’s choice of penalty kickers during the Euro2020 final is the most recent example of wrong decision made after an algorithm’s suggestion.
Coach Southgate revealed that he had chosen the players and their order based on the outputs of “artificial intelligence” (AI) algorithms; unfortunately, three of them failed to score their penalty and England lost the Euro cup to Italy.
Of course, there was no way to judge this decision “wrong” before the penalty kicks had started; nonetheless, it reminds us that predictions, suggestions and decisions based on data are inherently probabilistic and come with – sometimes big – uncertainties. This should warn against potential responsibility gaps.
Probabilities and statistics
The output of current AI algorithms is inherently probabilistic for several reasons.
To begin with, plugging some degree of randomicity in the algorithms helps making the output more general and useful. In addition, the informatic architecture usually includes – by design – some mathematical function that converts all the former calculations into a probability output. An image is classified as “cat” if it is highly probable that the machine classifies what’s being processed as a collection of features that use to belong to observed cats. I was purposefully cumbersome here. I could have written “if what the machine sees is a cat”, but it would have been inaccurate and probably wrong. This leads to the second point.
It is arguable whether machines “see” or “know”. What they do is to execute series of mathematical and logical rules.
Surely, these are often inspired and informed by the mathematical models of human perception and reasoning.
However, it is still unclear if such models are valid, true, exhaustive and unique – all features necessary to provide correct descriptions of reality.
Rotated images are known troublemakers and challenge the idea of “understanding”: unless accounted for during the initial development of the algorithm, rotating an object by some degrees might yield to misclassification.
Finally, there is the issue of causality. Without causal relationship among inputs and outputs, it is extremely complicated to distinguish between causes, effects and spurious connections. It is also impossible to speculate about “why” a result was obtained, and to test the effect of different interventions (counterfactual analysis). This results in poor explicability of the outputs and in further uncertainties.
Algorithmic causality
Because the philosophical debate around causality is extremely rich and variegated, I limit the present discussion to a working definition that can apply to AI algorithms.
If we can describe two (or more) real world phenomena with mathematical variables and say that one variable is a function of the other, we can then say that one phenomenon causes the other. The two are causally related. To validate the functional relationship is the main aim of science, that is, testing alternative hypothesis, verifying the predictions in unseen cases, stressing counterfactual examples.
On the contrary, if we observe the two variables behaving similarly, but there are still open questions about the interference of other variables, or their inter-relationship is unknown or doubtful, we can only conclude that the two are correlated.
Correlation is much weaker than causality. It can only tell us “how” the variables evolve, without hints about the “why”.
Spurious correlation is a classic case of observed correlation that must be complemented by some intuitive notion of causality.
Correlation Nicolas Cage and drowing in a pool
You might be impressed by the extremely high correlation between the number of films Nicolas Cage appeared in and the number of people who drowned after falling into a pool (incidentally, the correlation is higher than what’s observed between many gene effects and diseases!). However, this sounds absurd. Why should a person drown after hearing that Nicolas Cage filmed a movie? Or, on the opposite, is Mr. Cage so heartless to film movies after knowing that some person drowned?
There is clearly something wrong. “Clearly” comes from our inner notion of causality: despite evolving similarly, there is no way the two variables are connected by a causal relationship.
To solve the paradox, we need to invoke some extra piece of information, on top of pure statistics.
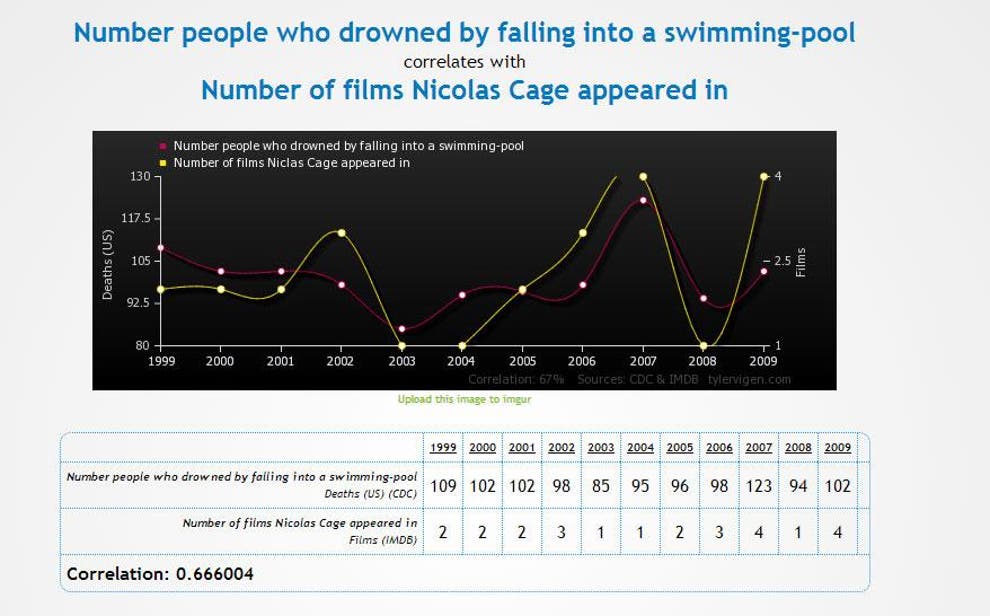
Including causal knowledge into algorithms is still not on the forefront of public debates, but an increasing number of scholars is being concerned.
Some argue that, without causality, we would never make another step towards real artificial intelligence: statistical correlation alone is just too weak and not representative of human thinking schemes.
Some algorithms that include such a notion are being developed, but they are still in the preliminary phase. Their promise is to provide explanatory power, develop better models, and allow testing counterfactual (“what if X had happened instead of Y..?”)
How this all translates into responsibility
The last decade has been characterized by surging interest on data and data-driven methods. In many cases – pattern recognition, image classification, art generation – these algorithms can automatically generate results that are very similar to humans’, hence driving productive activities as well as public perception on their potentials.
Given their increasing prowess, one might ask “to what degree can we use them to make decisions, and to take responsibility for them?”.
According to what discussed above, to quite a low degree. Data analysis and algorithms are amazing to complete known tasks, to unravel potentially useful connections, to inform, to provide new pieces to discuss, to stimulate hypothesis.
[algorithm and data analysis] come with severe limitations when used for automated decision-making.
It is a human choice to accept a probability output, and to look after suggested correlations.
And, because humans are able to create and justify causal relationships, they should take responsibility.
Coach Southgate offers an example. He fully acknowledged the potential of data and algorithms, he used them to inform the list of penalty kickers, but he did not back up when the strategy failed.
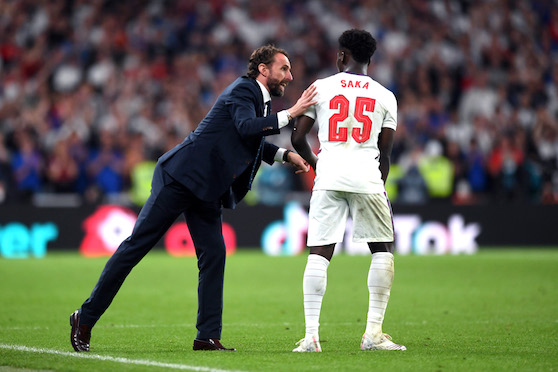
nella foto: Gareth Southgate-Bukayo Saka
He took responsibility on the choice. In uncertain and fluid scenarios, when there is no such a thing as “perfect forecasts”, past statistics are helpful to construct a decision base – but human intuition and sense of responsibility are not yet ready to be taken over.
- Director of Interdisciplinary Research @ House of Ethics / Ethicist and Post-doc researcher /
- Latest posts
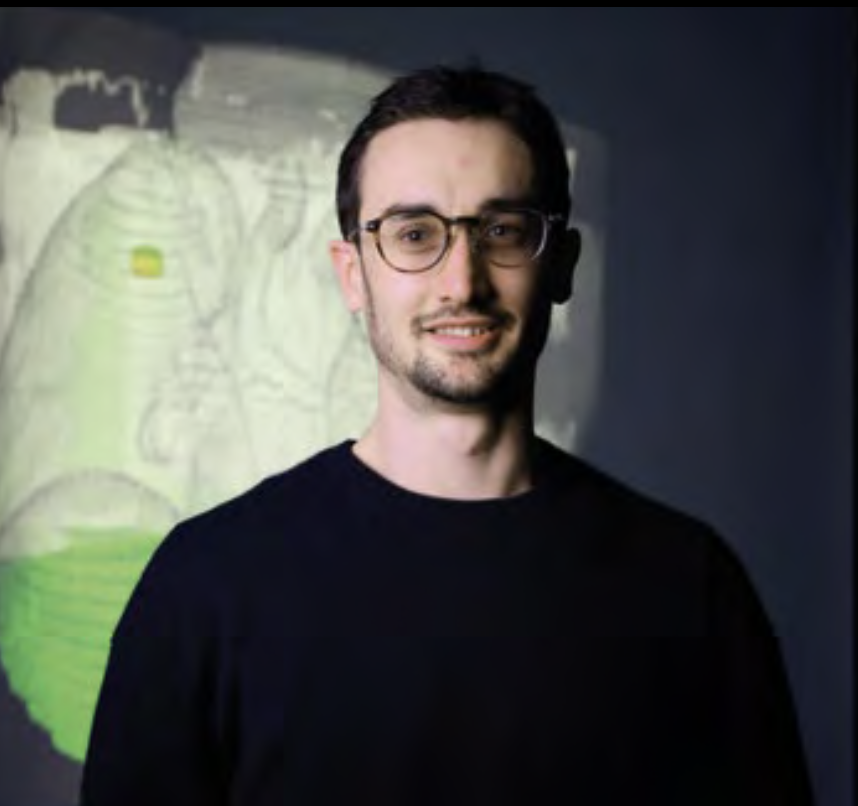
Daniele Proverbio holds a PhD in computational sciences for systems biomedicine and complex systems as well as a MBA from Collège des Ingénieurs. He is currently affiliated with the University of Trento and follows scientific and applied mutidisciplinary projects focused on complex systems and AI. Daniele is the co-author of Swarm Ethics™ with Katja Rausch. He is a science divulger and a life enthusiast.
-
In our first article of two, we have challenged traditional normativity and the linear perspective of classical Western ethics. In particular, we have concluded that the traditional bipolar category of descriptive and prescriptive norms needs to be augmented by a third category, syngnostic norms.