Run Forest Run: GenAI and the Productivity Glut
Run Forest Run: GenAI and the Productivity Glut
After OpenAI’s successful generative AI techno-putsch spearheaded by the release of ChatGPT, followed by a myriad of LLMs by competing Big Tech, followed by an avalanche of fine-tuned models, plugins, apps, APIs and more, the prompt-drunk (business) world has been promised to finally reach the prosperous Productivity Graal.
What a fantastic outlook if it weren’t for this annoying question by Nobel Prize in Economic Sciences Paul Krugman asked back in 1997: “Is Capitalism too Productive?”.
To explain the elephant in the room, Krugman introduced a heterodox radical economic doctrine called the “global glut”. Glut referring to overproduction, oversupply, and excess on the supply-side.
After multiple attempts to counterweight the failings, flaws, and regulatory temporary bans of an immature, non robust, privacy and IP violating technology, the Tech Titans finally pulled the productivity rabbit out of the generative AI hat. Abundantly capitalizing on Krugman’s 1994 motto
“Productivity is not everything, but in the long run, it’s almost everything.”
Eventually flipping the much hyped productivity genAI coin to discover the “productivity glut”, and finally the “Revenge of the Glut” as mentioned by Krugman in his 2009 NY Times op-ed.
In this article we analyze the technological genAI productivity glut in the light of Krugman’s economic global glut.
Inevitably, fundamental questions auto-generate when forensically focusing on the oversupply of, first genAI tools, and second those tools producing a content and data productivity glut.
What does productivity mean in the Age of generative AI? Does genAI make us more productive? When does genAI productivity become counter-productive? What is the revenge of the technological glut?
And finally, can genAI productivity be ethical?
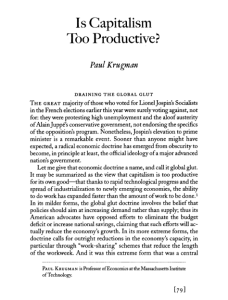
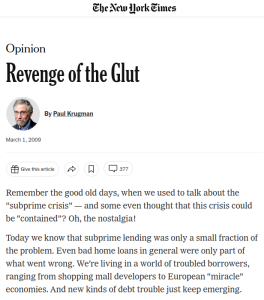
I. The GenAI Productivity Glut
No need to be a rocket economist to understand basic market dynamics. There is an offer, and there is a demand. Benefits and deficits result from differences between those two forces.
Productivity is defined as the ratio of what is produced to what is required to produce it. The relation between the resource and the result of a production, mainly in relation to labor productivity. One worker producing one output. Quite nice since computational generative AI works with inputs and outputs.
As of lately the productivity narrative has been spread like butter. Basically pushing the corporate and individual FOMO emergency buttons “If you don’t jump on the genAI speed train, your business has no future”; the same for individuals “If you don’t use these tools and become a prompt wizard, you underperfom and be less valuable to those using the tools”.
The productivity rhetoric hype
A quick search on Google gives an impressive list of “productivity boosting” headlines in relation to genAI.
Flanked by official productivity statements from market leaders or international institutions like the World Bank as recently stated by Jan Walliser, the Vice President of the Bank Group’s Equitable Growth, Finance and Institutions (EFI) practice group. His stressing on “accelerating growth” when talking about “bringing the most current advice to our clients about accelerating growth” is a top priority.
Some examples picked randomly on the Internet and social media, show how infiltrated and effective the rhetorical productivity machine is.
Playing on semantic confusion and ambiguous understanding of the concept of “productivity”
How scientifically accurate are these hyped productivity forecastings blistered to genAI tools?
Two observations are to be made: first, so far there is no sign of global productivity gains through genAI; second, there is a conceptual confusion about “productivity” serving the narrative. Even for a basic concept like penguin people differ in their understanding. Explaining why we often talk past each other; some accidentally, others intentionally.
Tech Titans, they use productivity in an ambiguous way as usually demonstrated and instrumentalized in political rhetoric.
When they refer too productivity of genAI, they refer to tools that are performance-based; however businesses and mainstream public extrapolate. Thinking and understanding productivity boom.
However, GenAI productivity, as of now, is only task-related, thus performance-based. This confusion is masterfully crafted into an “hallucinating productivity hype” narrative.
“Protracted productivity slowdown”
Erik Brynjolfsson, Stanford Professor talking at Microsoft, on “How AI Will Transform Productivity”
“I think these tools can and hopefully will lead to more widely shared prosperity. If we play our cards right, the next decade could be some of the best 10 years ever in human history.”
“Predictions about the economic impact of technology are notoriously unreliable.” Krugman
Any tech hype by Big Tech has been tampered by Krugman. According to the economist the rise of large language models (LLMs) like ChatGPT and Google’s Bard are unlikely to make any major economic impact just yet.
“History suggests that large economic effects from A.I. will take longer to materialize than many people currently seem to expect. That’s not to say that artificial intelligence won’t have huge economic impacts,” he concedes. “But history suggests that they won’t come quickly.There is a protracted productivity slowdown.”
“ChatGPT and whatever follows are probably an economic story for the 2030s, not for the next few years.”
To illustrate by comparison, Krugman referred to the rise in computing power during the mid-1990s and its lagged effect on labor productivity.
Examples of used productivity / performance rhetoric - all the same words
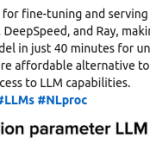
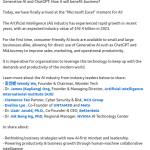
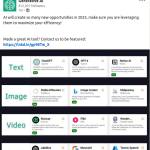
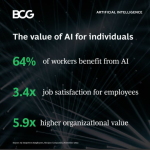
The production of glut
The other crucial concept radically ignored in the productivity equation is the flip side of productivity which Krugman calls “glut”.
With generative AI, the phenomenon went mainstream overnight at high-speed and at large scale. Too early, too fast, too much and everywhere. In excess.
The tools are used industry- and worldwide. We produce, and supply more content, than needed, or asked for. Thus the production of glut.
In relation to productivity, Krugman refers to “glut” as an effect of capitalism being too productive for its own good.
“Thanks to rapid technological progress and the spread of industrialization to newly emerging economies, the ability to do work has expanded faster than the amount of work to be done.”
What is glut?
The Merriam-Webster dictionary defines glut as oversupply and excessive quantity. Everything that is too much from a supply-side. Supply over-exceeding demand. An overflow, flooding, or excess of production.
Ideas of excessive productivity are not new. As Krugman points out postwar times were concerned with “automation”, replacing humans by machines. The global glut however remained dormant between 1950s to 1980s, which ironically coincides with the so called AI winter.
The Nobel Economist’s global glut doctrine holds three propositions
(1) Global productive capacity is growing at an exceptional, perhaps unprecedented rate.
(2) Demand in advanced countries cannot keep up with the growth in potential supply.
(3) The growth of newly emerging economies will contribute much more to global supply than global demand.
These three propositions hold true for the latest generative AI productivity glut. Terminologies coincide too. Semantics to describe the generative AI phenomenon are interchangeably borrowed from economics: growth, performance, capacity, unprecedented, emerging.
In the context of large language models, the notion of excess and over-dimensional volume is part of the denomination large language model. The amount of data needed to run these specific machine learning transformer models are humongous, and are expected to grow, even though Sam Altman claimed that the Age of Giant LLMs is already over.
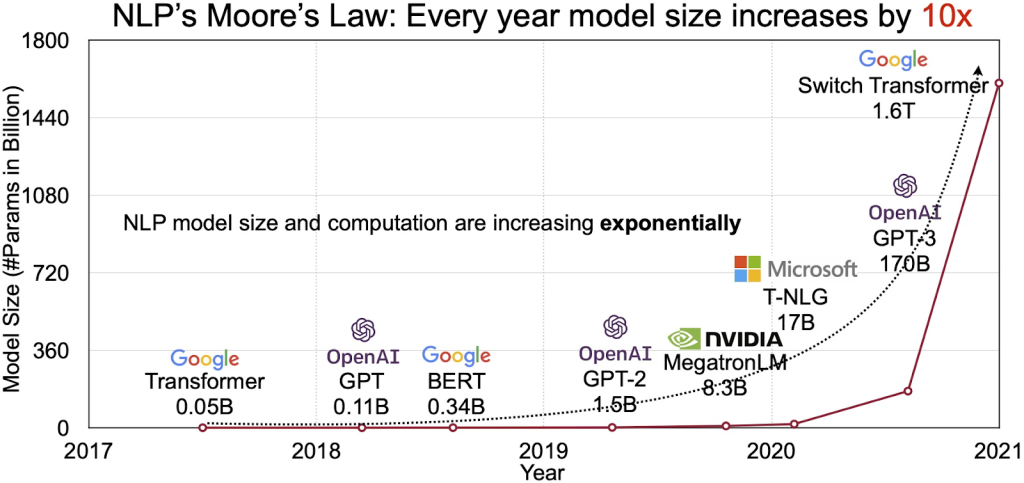
From productivity to GenAI productivity glut
In the case of generative AI, aren’t we facing a productivity glut rather than productivity itself? Glut being the revenge of purposeless productivity.
Only in April 2023, over 1,000 genAI tools were released. Headlines like “100 top AI tool to boost your productivity” or “generative AI such as ChatGPT and Midjourney to improve sales, marketing and operational productivity” or “It is imperative for organisations to leverage this technology to keep up with demands and productivity of the modern world” are flooding the market.
Numerous LLMs were released besides GPT. Llama, Alpaca (Stanford), Vicuna (University of California/Mellon/Stanford/Berkley, San Diego), Koala – fine-tuned on LLaMA (Berkley Research Institute), Nebuly (open-source of IchatLLama), Freedom GPT (open-source on Alpaca), ColossalChat – ChatGPT type based on Llama (Berkley), Dolly (Databricks), only to name a few… within one month.
In parallel, massive layoffs by Tech Titans have spurred competition and the unleashing of uncountable new tools by fired data scientists who created faster and cheaper models. No wonder we hear Sam Altman prophesying about the end of LLMs… Not because they are dangerous, ineffective or too expensive (what they are) but because of the smaller more competitive LLM glut.
What is the purpose of productivity?
“Enhancing productivity is essential to achieving sustainable enterprises and creating decent jobs – both core elements of any development strategy that places the improvement of peoples’ lives as its main objective.” as mentioned in the opening paragraph of Preface of “Driving Up Productivity” by the International Labor Organization, 2020.
As AI-generated “products” have grown exponentially, and highly overrun market demand; the over-production of content stands in no relation to the demand.
According to a March 2023 survey by wordfinder.yourdictionary.com of 1,024 Americans and 103 AI experts reveals that 41% of the interviewed admit they use the AI site to generate ideas, 11% use it to generate computer code, 10% use it to write resumes and cover letters, and 9% use it to create presentations.
These are quite low numbers for a national productivity booster in very selected fields of operation.
29% admitted that they’ve done it behind their employer’s back.
“Having a technology isn’t enough. You also have to figure out what to do with it.” Krugman
Traditionally the purpose of productivity was identified as being prosperity, growth, performance, competitiveness, revenue generation, profit, benefit, market capitalization, on a macro economic level. A level down, in business management, we find the concepts such as excellence, quality, effectiveness, efficiency, …
But glut is missing purpose, and genAI productivity is in need of a new purpose.
The self-serving and ethically void purposes as we have lately witnessed by ruthless leadership styles, irresponsible business strategies and unreliable promises for the “common good” need to be redefined. In the Digital Age where data-driven economies lie in the hands of a few, purpose urgently needs to be redefined.
II. The Revenge of the GenAI Productivity Glut
Glut is revengeful. Krugman’s economic impact of the global glut has been referred to the “Revenge of the Glut”.
“If you want to know where the global crisis came from, then, think of it this way: we’re looking at the revenge of the glut.”
The “introduction of improved technology might have some adverse effects”. This statement can easily be observed in a generative AI context. AI pioneer Geoffrey Hinton leaving Google to warn about AI risks is only one of many.
When does productivity become counter-productive?
We do see several scenarios: causing three levels of debt, intellectual, ecological and ethical.
Intellectual debt
The concept of “intellectual debt” was first introduced by Jonathan Zittrain in the New Yorker article “The Hidden Costs of Automated Thinking” in 2019.
Zittrain states that overreliance on AI may put society into intellectual debt. “As we begin to integrate their insights into our lives, we will, collectively, begin to rack up more and more intellectual debt.”
“A world of knowledge without understanding becomes a world without discernible cause and effect, in which we grow dependent on our digital concierges to tell us what to do and when.”
Ecological debt
It comes as no surprise that technology has been vastly energy consuming for years. It started with Big Data and cloud computing and data centers. In 2011 Greenpeace was issuing a widely hailed report “How dirty is your data? – A Look at the Energy Choices That Power Cloud Computing” with focus on IT carbon and energy footprint.
Followed by “Clicking Green” reports and their latest annual “Clean Cloud 2022 on ChinaTech” report.
LLMs however play in a totally different league. Researchers from the University of California Riverside and the University of Texas Arlington have shared the yet-to-be-peer-reviewed paper “Making AI Less Thirsty“.
Findings are shocking.
The paper reveals how the environmental impact of “AI training GPT-3 in Microsoft’s state-of-the-art U.S. data centers can directly consume 700,000 liters of clean freshwater (enough for producing 370 BMW cars or 320 Tesla electric vehicles).”
“ChatGPT needs to “drink” a 500ml bottle of water for a simple conversation of roughly 20-50 questions and answers”
Now consider ChatGPT’s billion users.
“Google’s LaMDA can consume a stunning amount of water in the order of millions of liters and newly-launched GPT-4 even that has a significantly larger model size.”
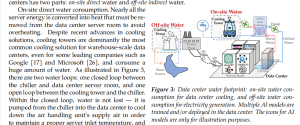
Ethical debt
The concept of “ethical debt” linked to generative AI technology has been introduced by Casey Fiesler. The ethicist rightfully insist on the responsibility of Tech Titans with societal harm that will hit later.
In her article “AI has social consequences, but who pays the price?” she explains the derivative form of “technical debt”.
“In software development, the term “technical debt” refers to the implied cost of making future fixes as a consequence of choosing faster, less careful solutions now.” (Fiesler)
Referring to the well-known ethical and legal concerns of AI systems amplifying “harmful biases and stereotypes, privacy concerns, people being fooled by misinformation, labor exploitation and fears about how quickly human jobs may be replaced”
Just as technical debt can result from limited testing during the development process, ethical debt results from not considering possible negative consequences or societal harms. And with ethical debt in particular, the people who incur it are rarely the people who pay for it in the end. (Fiesler)
We would like to go even further. Instead of only focusing on Tech leaders, users too bear responsibility. We as a society are responsible for our choices and actions.
We need to be clear about this. The intellectual, ecological and ethical debts are not just originated by Big Tech. It is our all choice to use or not use technological tools in excess, and at which purpose knowing the potentially harmful effects on humans and nature.
Ballooning human debts
The combination of the three dimensions of debt caused by the productivity glut of generative AI and AI will eventually cause a ballooning debt effect.
Similar to balloon mortgages, each level of debt is feeding and being fed by each other.
III. What does the genAI Glut look like?
After several months we are already observing and being impacted by the results of the genAI productivity glut.
Dark Data Glut
The sheer astronomical amount of daily prompted data, on a corporate and individual level, that is never read, listened to or seen is lost data. This data is never analyzed, remains unsorted nor used, and is just hanging in servers.
Useless but produced, and congesting space and using resources. Dark data.
Dark data is most of our produced data since generative technologies produce mainly unstructured data which is the most difficult to sort.
“By 2025, IDC estimates there will be 175 zettabytes of data globally (that’s 175 with 21 zeros), with 80% of that data being unstructured.
90% of unstructured data is never analyzed.” (source)
Needless to say that in the first month of ChatGPT being launched the amount of dark data must have grown exponentially with millions of prompters around the world playing with, testing and tricking the bot.
Even more bottles of water for storing that data. Besides producing it. This data productivity glut is massively impacting nature and boosting the ecological footprint. Green data has no place in generative technologies.
Decision data glut: Surrender to automation
The data glut has another effect on businesses, and mainly decision makers. The excess of data being available has a counter-productive effect on decision making.
The latest report The Decision Dilemma by Oracle focused on a crucial question: Is data helping or hurting?
72% admit the sheer volume of data and their lack of trust in data has stopped them from making any decision.
Data glut has a counter-productive psychological effect on executives, employees and users: feeling overwhelmed.
Data is hurting [people’s] quality of life and business performance.
Besides feeling overwhelmed, people feel under qualified to use data to make decisions. The consequence is that “overrun executives are most likely to hand decisions over to systems.” Because of Decision Distress and Stifling Success.
Decision Distress
- 85% of business leaders have suffered from decision distress.
- 83% of people agree that access to more data should make decisions easier, but
- 86% said that even with more data, they feel less confident making decisions.
Stifling success
- 91% of business leaders admit that the growing volume of data has limited the success of their organization.
In the very near future, most information on the internet will be at the best, average to bad information, misinformation, disinformation, fake news, deepfakes, polarized opinions.
With the hyper production of synthetic data, unprocessed data by generative AI will be hard to find.
Good ideas, original styles and researched insights will battle against copied and collaged texts.
Deep pocket customers looking for higher service
One potentially foreseeable consequence might be that a certain profile of people won’t use certain technologies anymore, and certain customers will prefer companies doing work differently.
Loyal costumers, and mostly deep pockets will prefer higher standards than the average gen AI products.
Good customers won’t accept to be spammed by computer-generated messages and look for higher service culture. Usefulness and accuracy will pay off against the glut of non-stop communication with synthetic purpose.
Glut in the production pipeline
meaning a part-of-the-process enabler. And that’s a major danger to businesses. Any CEO and executive manager does know the difference between process, task and skill.
Excessive generation of content, and proliferation of data might speed up parts of a process, but at a certain point slow down the global Chain of Production.
Generative AI only addresses a single task at a time T. In such a constellation the congestion caused by glut is highly dangerous.
As we have stated in a previous article co-authored with Daniele Proverbio on GenAI and Responsibility, the Chain of Accountability is valid for managing productivity glut.
Glut undergoes the same bullwhip effect as accountability does in a process of data flow.
Glut production does not occur on the entire pipeline. It is localized since related to specific tasks. However it affects the entire pipeline.
And the danger of productivity glut is real with GPTs and LLMs plugins or APIs.
Local glut congestion affecting the entire process will effect the entire cost-benefit calculations at large scale.
One example of productivity glut hindering and slowing down the initially high speed process has been observed in biosynthetic labs as shared in the article “Does automation do more harm than good” by Adi Gaskell.
“The promise is that AI will enable scientists to both massively scale up their work while also saving them time that they can then devote to other tasks. The reality, however, was not quite so clear-cut.”
There was a significant increase in the number of experiments and hypotheses that had to be performed, with automation amplifying this increase. While on one hand, this allowed more hypotheses to be tested and a greater number of tweaks to the experiments to be made, it also greatly increased the amount of data to be checked, standardized, and shared.
To this adds the ethical and legal problem of accountability in case something goes wrong. The speed might turn in a financial shortfall and production lag.
Scams, privacy and confidentiality risks
With the 24/7 productivity of generative AI, another kind of production glut will skyrocket: scams.
The tidal wave of individual scams and corporate cybersecurity risks is largely undervalued in the productivity hype. Repairing costs, legal bans will explode in scope and scale.
Needless to say that LLMs or LlaMA will run fast and cheaply on scammers’ laptops and “can now run hundreds or thousands of scams in parallel, night and day, with marks all over the world, in every language.” as described in the article “Brace Yourself for a Tidal Wave of ChatGPT Email Scams”.
Another mostly over-looked pitfall of the productivity hype is confidentiality. Its negative reputation and cost effects on business and customers remain unveiled. JPMorgan Chase (JPM), have clamped down on employees’ use of ChatGPT due to corporate compliance and privacy concern touching on their core business. Whereas PWC announced its deploying of a chatbot service for 4,000 of their lawyers in over 100 countries to gain steam, with plans to expand this technology into their tax service.
Magic Circle law firm Allen & Overy “partnered with a startup backed by ChatGPT creator Open AI to introduce a chatbot intended to help its lawyers with a variety of legal tasks”. Insisting simultaneously that their fees would not be lowered for customers. (!!!)
The Magic Circle firm Allen & Overy has rolled out the tool – named Harvey and described as a “game-changer ”delivering unprecedented efficiency and intelligence.”(!!!)
Consider this: if the data you input in ChatGPT or any LLM is being used to further train these AI tools then you serve your data to somebody else. And if that somebody else is your competitor’s super skilled prompt engineer capable to extort information from the box, well…
Especially for the much hailed and claimed ease of writing up meeting reports, review contracts, composing company emails or any organizational time-consuming task.
We enter a new age of “industrial prompting espionage” at large scale and at low cost.
You might read OpenAI’s AI safety statement (similar to Google’s Bard and other LLM developers statement to AI safety) very differently now.
“We don’t use data for selling our services, advertising, or building profiles of people — we use data to make our models more helpful for people.”
The following matrix by the HBR gives guidance to low and high demand and risk in using GenAI tools. You notice that most medium level but wide tasks are high on demand and low on risk. All skills requiring critical thinking, higher expertise, confidentiality, accuracy and veracity are in the high/high quadrant.
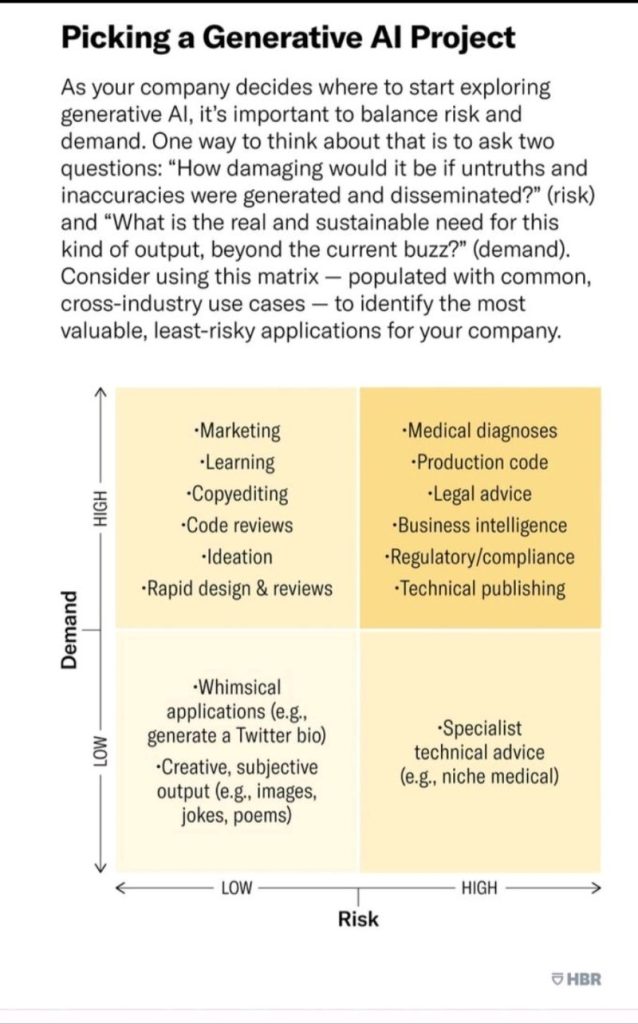
IV. Can Productivity be ethical? Yes, with purpose.
What if the genAI phenomenon’s quick adoption does not testify of the effectiveness of the system but rather of the ineffectiveness of companies. That most market actors fail and fall in setting sustainable and competitive purpose.
Most machine-learning systems don’t uncover causal mechanisms. They are statistical-correlation engines. Thus we need to focus and revalue the WHY, the purpose.
In times of GPT, general-purpose technology, purpose becomes key. Long-term revenue generation might be found in compensatory fields to technology where brand strategy all started. Employee loyalty, customer loyalty, quality management instead of risk management.
Thus it is imperative to set corporate purpose straight. Calibrating it and getting out of the computational tunnel. Questions to be asked are
Which values are linked to productivity?
What is the human purpose of productivity?
The time is ripe for responsible leaders and executives to redefine productivity.
“Optimizing for what?” The importance of purpose.
Just recently this symposium organized by Columbia University hit the nail. It went back to the basics by asking the most important question: “Optimizing for What?”
Going deep and beyond market metrics. That’s where ethical productivity steps in.
Ask questions such as “Is our productivity value-adding?” Does it create opportunities and translate into meaning for people and society?”
Because that’s what both employees and customers want.
Translate ethical benefits into financial profit by assessing purpose around three layers of ethical profitability: carbon productivity, social productivity and people productivity.
Carbon productivity: sustainability and care
In the article “What if we measured the thing that matters most: “carbon productivity”, the Australian David Peetz argues that people want economic growth and ecological survival.
ESG has been one of the hottest topics in the last years. However very few companies are champions. The outdoor apparel brand Patagonia has been radical in its corporate choices towards embedding sustainability and making customers proud to wear its cloths. Or the Finnish lifestyle and home furnishing design brand Marimekko. Both have embedded sustainability in products and minds.
Social productivity: purpose and responsibility
Social productivity is the response to the question: what does my product, brand or service add to people’s life? What is the value of our business?
Here prevail ethical concepts such as fairness, equity, inclusiveness, and care. Ethics of care is the more practical approach to social productivity at its optimum.
Shared responsibility towards society and duty of care are driving forces of new age productivity.
People productivity: performance, trust and empowerment
Building strong people brands are key to people productivity. Choosing a people-centric and customer-centric approach versus a performance-centric path has been a game-changing strategy for Airbnb. They achieve their first-ever FY profitability of $1.9B in 2022.
While performance strategies are important for driving revenue growth, brand building is crucial for enhancing emotional connections with customers, and establishing long-term profitability.
With generative AI all is put on people performance and computational trust. That equation is flawed because people are not even performant. The machine is performant.
The productivity narrative attached to genAI leads low-level and average leaders to be more concerned with performance metrics.
Forgetting that trust is essential for driving performance and creating a positive work culture.
The following video explains the benefits of trust versus performance.
Psychological safety erosion and AI anxiety are resulting brakes to performance.
For many executives this new genAI turn might be a wake-up call to reassess their own brand, product and employees worth and values in order to define a sustainable and ethical productivity roadmap. They will run their run, with purpose.
Insights by
Dr. Ingrid Vasiliu-Feltes and Cary Lusinchi, FHCA
Dr. Ingrid Vasiliu-Feltes
Deep-tech Entrepreneur
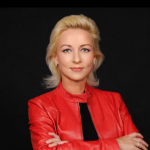
Generative AI has immense potential to transform education, learning, workflows and productivity. However, I do not endorse either one of the the radical positions that either aim to prevent adoption or to encourage chaotic, large-scale adoption of this technology without adequate risk analysis and proactive digital ethics programs in place.
It is imperative to have a balanced and responsible approach that prioritizes ethical principles and robust data governance. Although Generative AI can improve the speed of our execution in specific pre-trained tasks and increase our efficiency, it is highly dependent on valid and authenticated datasets, requires specific instructions to deliver expected results and a high level of human expertise to evaluate the veracity of outputs.
It is also crucial to ensure that it does not compromise privacy or amplify pre-existing biases. Hence, I advocate for a responsible, transparent, accountable and trustworthy generative AI deployment with proactive, tight digital ethics guardrails in place. Hybrid augmented intelligence, which combines the strengths of both humans and machines, is poised to transform industries and revolutionize the way we learn, educate and work.
However, to fully realize the potential of this technology, it is crucial to implement robust data governance and strict oversight of human-computer virtual workflows to avoid ethics and cybersecurity breaches. This requires optimal executive cognitive functioning and ethics by design for all human-computer-human interactions. When deployed in compliance with digital ethics and data governance frameworks, Hybrid augmented intelligence (HAI) can leverage the complementary strengths of human and machine intelligence.
Robust data governance is essential for the ethical and efficient use of HAI. This includes ensuring data consent, authentication, validation, and provenance. In addition to data governance, it is important to have strict oversight of human-computer virtual workflows to avoid ethics and cybersecurity breaches.
It would also require that all algorithms deployed are transparent and explainable, so that decision-making processes are clear and understandable.
To ensure optimal executive cognitive functioning, it would be essential for all team members involved during any of the Gen AI life cycle stages to have a strong understanding of the potential benefits and risks of HAI, as well as a deep understanding of the capabilities and limitations of the technology.
It is also important to nurture a culture of ethical decision-making, with clear ethics guidelines, ethics operational procedures, ethics KPIs, and ethics metrics. Implementing an “ethics by design model for HAI” at every layer and every step is critical for ensuring that all human-computer-human interactions are conducted ethically and with the highest level of integrity.
Cary Lusinchi, FHCA
Founder @ www.aiauditor.com
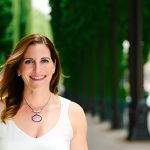
Generative AI alone won’t provide productivity that delivers real, measurable ROI value. Right now the average worker who is interfacing with ChatGPT-4 can perform single tasks at a time. For example, you can only ask a question and get a response/output before it needs more manual human direction and intervention (aka prompting).
We’ve all experienced how generative AI tends to breakdown and degrade in performance/accuracy when you provide it multi-step tasks or a chain of complicated inter-related questions.
Measurable enterprise productivity gains come with scaled automation. AutoGPT, an recently released open source application that leverages GPT-4 might be generative AI’s automation transformation.
AutoGPT divides complex multi tasks into smaller ones and then spins of independent instances in parallel processing to work on them.
They are the ‘new and improved’ project managers as they coordinate-schedule the work tasks, compile the tasks and deliver the result.
AutoGPT can be 100% automated, means it is empowered to complete tasks without any human intervention or guidance. And it has long term memory management (unlike ChatGPT However, autonomous recursive AI agents (AutoGPT) are only powerful as the access you give it through an API. Yes, we might have magical productivity gains via AutoGPT’s autonomous agents (will project managers go extinct?) but at what costs.
And are the costs really worth the gains?
What happens when you remove frequent and deliberate human interaction/agency from prompting workflow?
What automation biases magnify when autonomous planning & decision making happens at scale?
Who is responsible/accountable for evaluating/testing AutoGPT’s work?
When APIs are poorly or hastily, integrated into AutoGPT, vulnerabilities are plentiful; how many attackers will gain access to PII and sensitive data or execute other malicious actions?
Katja Rausch is specialized in the ethics of new technologies, and is working on ethical decisions applied to artificial intelligence, data ethics, machine-human interfaces and Business ethics. Katja is a linguist and specialist of 19th century literature (Sorbonne University). She also holds a diploma in marketing, audio-visual and publishing from the Sorbonne and a MBA in leadership from the A.B. Freeman School of Business in New Orleans. In New York, she had been working for 4 years with Booz Allen & Hamilton, management consulting. Back in Europe, she became strategic director for an IT company in Paris where she advised, among others, Cartier, Nestlé France, Lafuma and Intermarché. The proposed concept of swarm ethics evolves around three pilars : behavior, collectivity and purpose Away from cognitive jugdmental-based ethics to a new form of collective ethics driven by purpose.
For over 12 years, Katja Rausch has been teaching Information Systems at the Master 2 in Logistics, Marketing & Distribution at the Sorbonne and for 4 years Data Ethics at the Master of Data Analytics at the Paris School of Business.
Author of 6 books, with the latest being "Serendipity or Algorithm" (2019, Karà éditions). Above all, she appreciates polite, intelligent and fun people.