“Explain Explainability” or why AI Narrative does matter. About words, terms and notions used and misused.
Definitions. Terminologies. Explanation. Words.
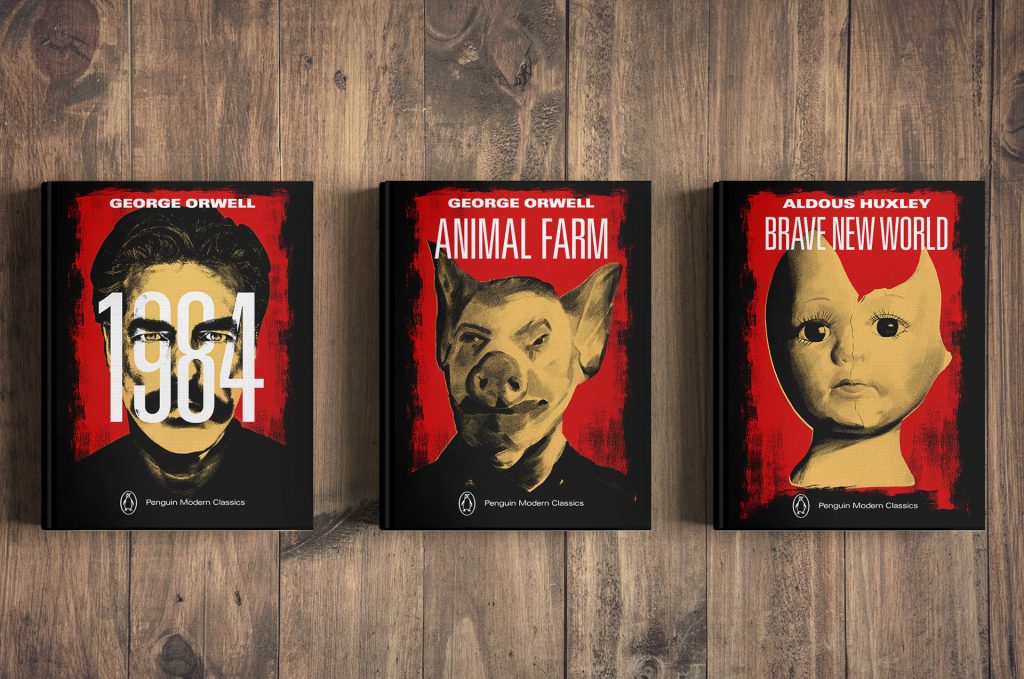
"Explainability" - please explain!
Explainability has become a word à la mode. However it is one of the most confusing, unexplained words in the high tech industry where understanding and transparency are key.
In the leatest EU proposal for an AI Regulation Explainability and Interpretability are put forward as a panacea to confusion, mistrust, blurred corporate and user visions.
Both notions should address the pressing concern of algorithmics bias, data integrity, and high-risk / harmful AI.
Since words shape our reality, the Narrative on and around AI has become paramount.
Explaining and understanding
In this article we won’t focus on the technical side of explainable models but on the linguistic and cognitive levels.
Already on a very basic level, in press articles and research papers, misplaced, misused and misinterpreted terms are common.
They have infiltered our daily speech and thinking patterns so that we don’t even question them anymore.
They are to be found in each language. We just scanned French, English, Italian and German articles and texts randomly.
Here are a few exemples.
- Will computers decide who lives and who dies? (Eastern Focus)
- Should computers decide who gets hired? (The Atlantic)
- A face-scanning algorithm increasingly decides whether you deserve the job? (Washington Post)
- AI-learns-to-write-its-own-code-by-stealing-from-other-programs
- L’intelligenzia Artificiale sente la depressione nella voce
AI chooses…
“Amazing AI robot learns the consequences of its own actions”
Implies that the AI robot has a certain degree of consciousness and is capable of any cognition. Amplified by “Amazing” AI robot.
Plus, the wording “Consequences of its own actions” leads to total confusion by misplaced logic and semantics.
The computer has no idea of “consequences”.
The processing of data is by no means an intentional action of the computer.
“What is an algorithm? How computers know what to to with data”
This title implies that WEdon’t really know what to do with data but the computer does. Especially with “black box” algorithms, the computer “knows” it all. Totally misleading. And wrong!
“AI learns to write its own code by stealing from other programs”
To steal (definition by Merriam Webster): to take the property of another wrongfully and especially as a habitual or regular practice.
Stealing is a conscious action.
Totally inaccurate and misleading in this context.
Definitions, roots, pioneers
Etymologically to explain derives from the Latin explanare “to make clear, make plain”, literally “flatten, to spread out”.
An individual fact is said to be explained by pointing out its cause, the why something happens. How and what.
The difference to interpretability. A (non-mathematical) definition by Tim Miller (2017) is: Interpretability is the degree to which a human can understand the cause of a decision. Another one is: Interpretability is the degree to which a human can consistently predict the model’s result. The higher the interpretability of a machine learning model, the easier it is for someone to comprehend why certain decisions or predictions have been made. A model is better interpretable than another model if its decisions are easier for a human to comprehend than decisions from the other model.
The Memorandum to the proposal for a EU-wide regulation on AI starts in medias res
The most recent Conclusions from 21 October 2020 further called for addressing the opacity, complexity, bias, a certain degree of unpredictability and partially autonomous behaviour of certain AI systems, to ensure their compatibility with fundamental rights.
Avoid opacity, ambiguity, unpredictability. Be clear. Concise, accurate, simple but not simplistic.
Heinz von Foerster’s and Joe Weizenbaum’s take on AI Narrative
For von Foerster, not the process is the common denominator between a computer and humans, but the language or coding.
Language and coding have the same structure. The same syntax. However there is no semantics for computers.
That’s why a computer separates and we choose.
We choose. The computer separates. (Heinz von Foerster)
The computer separates and organizes the sensory stimuli or sensor data in an order system defined by the programmer. Eventually creates new ones.
Von Foerster distinguishes the brain completely from other “non-trivial” machines that perform cognitive processes.
For Von Foerster, a thought process must have 3 qualities – perception, remembering, deciding. If one falls away, the system is without cognition.
The computer makes a difference, a human decides.
Data processing in the human brain takes place by distinguishing and classifying various sensory stimuli into an emerging (or learned) category system.
This aggregated view by the brain forms the basis for decisions that leads to conscious action. Our actions are intentional!
Difference between human and machine cognition
When describing the performance of a computer, Heinz von Foerster knowingly used appropriate terms, especially verbs, related to technical processing.
The data processing in the human brain, in contrast to that in the computer, is not explained in a purely formal logical way, but through the ability of the decision taking that enables the possibility of a founded interpretation.
Only knowledge, in the form of individual and institutional recognition of the criteria of production, can assess the transition from data to information and consequently decide which realities (signals, data) can become realities (information, knowledge), which in turn are relevant to world-generation. (Heinz von Foerster)
There still is a difference between learning and understanding. Even with us humans. A diploma or a title does not mean skills!
There is Machine Learning. However it is far from Machine Understanding!
In “Computer Power and Human Reason – From Judgment to Calculation” (1976), Joe Weizenbaum writes
“The computer can close according to logical laws or between 0 and 1,
Distinguish, separate and classify and thus generate new logical knowledge in the computer.
The computer, however, can not choose and evaluate, thus generate relevant new knowledge without the interpretation of humans.”
When it comes to language and cognition, one of the most elucidating experiments has been developed by John Searle.
The Chinese Room Experiment clearly shows the differences between coded computor language based on syntaxical rules and the human language with its words and semantics.
Figures on Explainability
The ultimate goal is “preventing harm”. Unfortunately, this malevolent take on defining the latest goal of AI is rather bothersome. It means that we are already far into harming.
In a recent article “Biden’s AI czar focuses on societal risks, preventing harm”
“There’s an increased need for education and training so that people know how to use AI tools, they know “sort of what” the capabilities are of AI so that they don’t treat it as magic,” Lynne Parker.
We see that the wording used by the US top “explainer” is extremely blurred – “sort of what” and “magic”.
Explaining needs concise, clear, distinct words. There should be no room for doubt. However here we create new room for doubt.
Three main inhibitors to AI have been identified : technical, people, and ethical.
A recent survey by Juniper provides astonishing results in regards to “explainablity” in the sense of “understanding”…
The question of AI Governance and getting people, employees and users, onboard is most revealing. Nearly all interviewees agree on the importance of AI Governance – 87%.
67% of respondents reported that AI has been identified as a priority by their organizations’ leaders for a fall 2021 strategic plan, and 87% of executives agree that organizations have a responsibility to have Governance and Compliance policies in place to minimize negative impacts of AI.
Yet it is their lowest priority by 7%!
Yet only 7% of executives said they haven’t identified a company-wide AI leader to oversee AI Strategy and Governance.
There is a dawning discrepancy between the objective (what) and the implementation (how) to do/say/word/explain it.
Further research found that organizations scaling their AI capabilities, by integrating employees into solutions and raising user satisfaction has been identified as a difficult task.
73% of organizations struggle with the preparation and expansion of their workforce to integrate with AI systems.
That’s an explainablity issue.
The explainabilty goals are to be considered on two levels:
- to train or hire people to develop AI capabilities within an organization
- TO/AND train end-users to operate the tools themselves
Source: Juniper surveyed 700 IT global decision makers who have direct involvement in their organization’s AI and/or machine-learning.
The Chain of Explainability
High-risk algorithms need explainability as described in the recent proposal for an EU-wide AI Regulation.
Transparency is intrinsiquely linked to explainablility. However, these two concepts differ.
Transparency adds to the explainability but does not strictly induce it.
AI algorithms have different levels of opacity. The highest level of opacity is to be found in neuronal net algorithms – the so called “black box” algorithms.
Technical explainability has become a discipline by its own – XAI. The ultimate goal being to create “glass box” models, completely transparent and explainable models.
What is the difference between interpretability and explainability ?
Interpretability is the ability of a model to make anybody understand the input and output. The cause and consequences.
Explainability on the other hand goes a step further and gets more into the parameters of transparency.
The algorithm which has been the most interpretable and simplest for human understanding is the decision tree. All the linear models are innately interpretable. That’s the technical side.
What about the linguistic side?
How to translate concepts like explainability or interpretability into a fully comprehensive language for end-users, patients, children, elderly?
The European Parliament published its initial revisions to the planned Digital Services Act. Christel Schaldemose, the Danish MEP who will pilot the initiative through the Parliament, has put algorithm accountability on her priority list of reforms.
The EU executive also requested that online platforms open up their algorithms and illustrate how they are triggering disinformation. It will be debated on 21 June and MEPs will be able to submit their amendments until 1 July 2021
Is easy-to-understand, easy-to-explain? NO!
Even if a technical model can be easy to understand, yet the outcome might be non-transparent and difficult to shape into words.
Over the years, companies have always been faced with issues of explainability. In the advertising industry, the most difficult part is not the creative side but the initial brief. To properly explain an advertising campaign to an agency.
Most briefs were badly formulated, too many generic words used and ideas poorly expressed. The result were mediocre campains and huge money losses.
The Chain of Explainability
For AI the stakes are higher. The focus on an explainability method needs to be taken seriously.
How to successfully translate the idea of transparence, and interpretability of a model into a concise and understandable message?
Companies need to identify their own the Chain of Explainability. The Chain of Explainability can add significant value to the Chain of Value for the Company.
There are at least three different levels in the Chain of Explainability
- UPSTREAM : The designers/developers – with techno-pratical language
- => technics > communication
- MAINSTREAM : The employees of the company – hybrid techno-communicative language
- => technics + communication
- DOWNSTREAM : The end-users – depending on their nature communicative language teinted with technics
- => communication > technics
The nature of the Chain of Explainability is not linear. It is intertwined and co-feeding itself. In order to maximise the power of the narrative, each level needs to be coherent, comprehensive and complementary.
XAI or AIX?
XAI - explainable AI - or explain AI?
Explaining is about understanding, about meaning, about cause, about purpose.
Beyond the technical explanations, the narrative needs to be meaningful and relatable.
Why is it so important?
Because of the misleading and too often self-formulated narrative served by the Big Tech that sounds like”all of this is amazing and fantastic, and we shape your future.”.
But do I want them to shape my future? Do I see the future like they see it? With digital divide, biases, discriminations, dubious HMI, power inequities. Maybe I have higher standards than the ones they feature.
Like Kate Crawford writes in her book The Atlas of AI
“We’re looking at a profound concentration of power into extraordinarily few hands.”
That means shifting away from just focusing on things like ethical principles to talking about power.
Talk about Power
Talking about power, yes! But then we need powerful words. We need a powerful narrative. We need to be clear what is distopian and what is utopian. We need to be clear what is valuable for us and what clearly is not.
We need to demystify the artificial starification system of AI where silly and insignificant performances suddenly rise to stardom status and gain a disproportionate importance.
In any kind of supremacy system, words, images, communication techniques, populist propaganda was an important instrument to manipulate and mislead people. To intimidate. To divert trust into the hands of a few.
That’s how words can fight for power. A clear and accurate narrative,a well-trained explainability technique could counter-balance a self-declared leading system and even prevent this system from becoming abusive.
In literature we certainly do think about George Orwell, Aldous Huxley, Capek, Asimov…
Once you properly call things by their name, the “soufflé collapses”, the system goes down. Words can heal and prevent harm. The right words.
Explainable AI is needed. Yes. Certainly. But before having explainable AI, AI needs to be properly explained…
AIX first, then XAI
Nowadays eager readers find the equation XAI = FAT.
Even FATE, by adding the ethical component for AI Governance.
FAT stands for Fairness, Accountability and Trust. However one can only reach these objectives by explaining and nuturing understanding.
The linguistic/rhetorical side as well as the cognitive side of explainability being key..
WHAT needs to be explained (issue, problematic)
And
HOW! (structure + wording)
1. Fairness: Explain how model outcomes are without any discernible bias. That’s the what. 50% of explainability.
However how to fomulate it into understandable words for different audiences (non-tech, children, elderly…) is the crucial part of explainability.
2. Accountability: Explain model behaviour and outcomes. Ok. But then the crucial part starts. Transmit and translate the message.
Put it into understandable, relatable non-tech words for people to grasp to importance and purpose.
3. Transparency: How the model works internally and predictions are made. That’s on a technical side already a challenge.
In a second move, putting it into easy concepts and words yet another challenge.
“Explainable algorithms” do already exist. But these are not the solution we need.
We need the rights ideas translated into the right words to be rightfully understood.
“Explainability and interpretability are about meeting the quality standards we set for ourselves as engineers and are demanded by the end user,” says Kostas Plataniotis, a professor in the Edward S. Rogers Sr. department of electrical and computer engineering in the Faculty of Applied Science & Engineering.
“With XAI, there’s no ‘one size fits all’.” You have to ask whom you’re developing it for. Is it for another machine learning developer? Or is it for a doctor or lawyer?”
Formalizing vs formulating
A tech narrative, an employee narrative and a user narrative.
How to avoid to turn XAI, which is designed to be a technical “glass box”, again into a cognitive and linguistic “black box” by bad and inaccurate explaining.
Technical transparency can be hinderd by bad communication, mediocre linguistics and faulty cognitive understanding by the next-on-line explainability chain agent.
The first is predominently technical; the second one explicative, semi-technical and communicative, in an easy to understand yet informative style; the last one, very informative and simple words, but not simplistic.
How to properly explain in easy-to-understand words technical concepts to non-tech people?
How to explain in understandable words that an XAI algorithm can run simultaneously with traditional algorithms to audit the validity and the level of their learning performance?
How to explain that this new XAI approach provides opportunities to carry out debugging and find training efficiencies?
How to word the problem and intent of a particular scenario that always requires adjustments to the algorithm – to interpret and explain the “heat maps” or “explanation maps” to a medical professional and to a patient?
These are just 3 examples that would need to be rephrased in a different “explainable language”.
What makes a good explanation?
What makes a valuable interpretation?
Both involve a certain skillset. Cognitive and linguistic skills.
A good explainer, technical communicator needs three particular qualities
- to be able to actively listen
- to be able to analyze and quickly understand
- to formulate adequately
In order to succeed in this task one needs an extensive vocabulary, precise and accurate words to avoid misinformation, distorted interpretation, and false transmission.
Not just descrptive qualities but also linguistic forecasting in order to prevent any misunderstandings or falsifications. Preventive linguistics that is “misunderstandable-proof”.
Skills of a good AI Explainer
Seamless communication involves understanding and communicating with people who speak different languages.
At the cross roads of computational linguistics, cognitive linguistics and communication. The AI Explainer is a hybrid communicator with sound technical expertise and knowledge.
An active listener
Not just listen but proactivey listening and checking upon the accuracy. This involves asking questions. The listener must be able to fully comprehend the system, model, purpose or goal. On different cognitive and linguistic levels.
Well informed on market trends and IT savvy
As a good explainer you need to juggle between multiple languages in a limited time frame, it is important to have good command over the languages. Good vocabulary and quick structural qualities of ideas.
Good writing and speaking skills
Besides having a good grasp on the linguistic skills, a successful explainer should have good writing skills to understand the local culture and idiomatic importances.
Adheres to the Code of Ethics
No compromise with ethics. If terms are inappropriate, flaws are being detected, the explainer/interpreter must raise the issues. If words are simply misleading they should not be used.
Wrong words can cause as much harm as a high-risk AI. They trick and manipulated people into a wrong understanding.
Overrating and underrating should be avoided. Integrity is key.
Vivid Mind
The explainer must be capable of connecting quickly the right information. AI might be connected to healthcare, ESG, Fintech, Mobility, Morality, Logistics, Arts, Law…
Stakes need to be understood and translated into accurate wording.
It is not about formalizing but formulating.
Explicit. Distinct. Clear.
- Founder HOUSE OF ETHICS
- Author's Posts
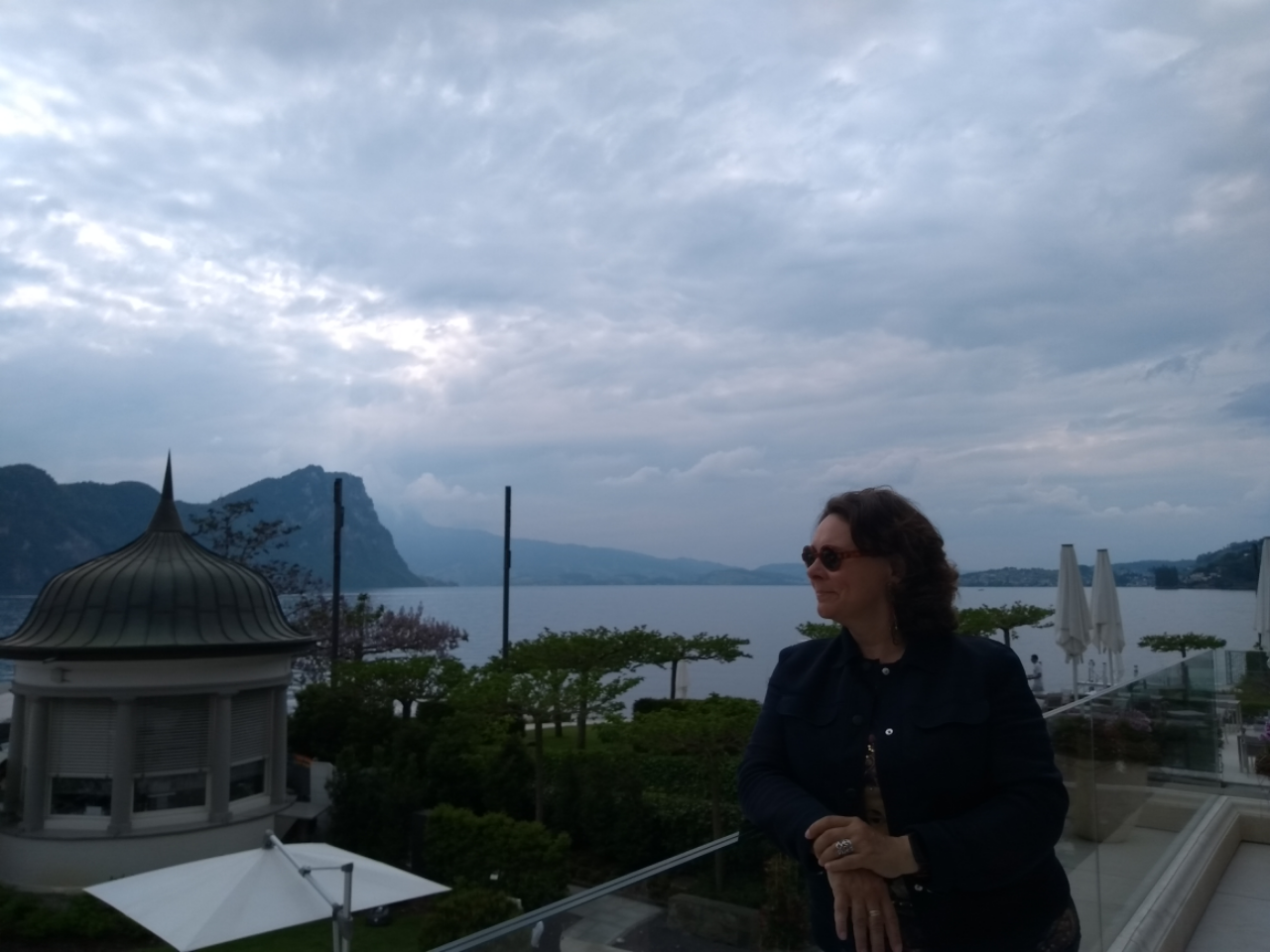
Katja Rausch is specialized in the ethics of new technologies, and is working on ethical decisions applied to artificial intelligence, data ethics, machine-human interfaces and Business ethics.
For over 12 years, Katja Rausch has been teaching Information Systems at the Master 2 in Logistics, Marketing & Distribution at the Sorbonne and for 4 years Data Ethics at the Master of Data Analytics at the Paris School of Business.
Katja is a linguist and specialist of 19th century literature (Sorbonne University). She also holds a diploma in marketing, audio-visual and publishing from the Sorbonne and a MBA in leadership from the A.B. Freeman School of Business in New Orleans. In New York, she had been working for 4 years with Booz Allen & Hamilton, management consulting. Back in Europe, she became strategic director for an IT company in Paris where she advised, among others, Cartier, Nestlé France, Lafuma and Intermarché.
Author of 6 books, with the latest being “Serendipity or Algorithm” (2019, Karà éditions). Above all, she appreciates polite, intelligent and fun people.
-
The proposed concept of swarm ethics evolves around three pilars : behavior, collectivity and purpose
Away from cognitive jugdmental-based ethics to a new form of collective ethics driven by purpose.